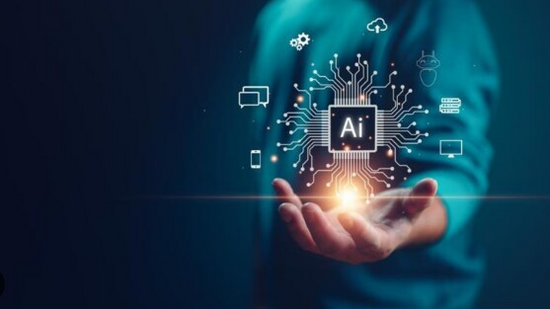
In a world increasingly driven by data, speed, and intelligent automation, traditional computing paradigms are no longer sufficient to keep up with the real-time demands of modern applications. Enter Edge AI, a game-changing approach that fuses the power of artificial intelligence with decentralized computing at the edge of the network.
From autonomous vehicles to industrial automation and precision healthcare, Edge AI is unlocking real-time intelligence in environments where every millisecond counts. But it’s not just about speed — it’s about resilience, scalability, efficiency, and privacy.
Let’s explore how Edge AI is revolutionizing decentralized computing and powering the next generation of real-time applications.
🌐 What is Edge AI?
Edge AI refers to the deployment of AI models and processing capabilities on local devices — sensors, gateways, smart cameras, smartphones, drones, and more — rather than relying solely on centralized cloud servers. The idea is simple yet powerful: bring intelligence closer to where data is generated and decisions are needed.
This means AI-powered inference and actions can happen at the edge, reducing the latency and bandwidth requirements associated with constant cloud communication.
🔄 Decentralized Computing: A New Architecture for a New Era
Decentralized computing distributes processing, storage, and control across multiple nodes, instead of centralizing everything in a single data center or cloud infrastructure. It enables systems to operate more autonomously, securely, and efficiently — particularly in disconnected or latency-sensitive environments.
Edge AI is a natural complement to decentralized computing. Together, they create a distributed ecosystem where data is processed locally, decisions are made on-device, and central coordination is minimized — all while maintaining scalability and intelligence.
⚡ Why Real-Time Applications Need Edge AI
In industries where timing is critical, even milliseconds of delay can lead to lost opportunities or dire consequences. Here’s how Edge AI meets the unique demands of real-time applications:
1. Low Latency Decision Making
Real-time systems need to process and respond to events almost instantly. By performing inference at the edge, devices can act on data without the delay of sending it to the cloud and waiting for a response.
2. Resilience in Connectivity Gaps
In remote areas, mobile environments, or mission-critical operations, reliable internet connectivity isn’t guaranteed. Edge AI ensures that systems can continue functioning intelligently even when offline or partially connected.
3. Bandwidth Efficiency
Constantly streaming raw data to the cloud is expensive and impractical. Edge AI reduces bandwidth usage by processing data locally and only sending essential summaries or anomalies for central aggregation.
4. Enhanced Data Privacy and Compliance
Processing data on the device helps safeguard sensitive information, especially in fields like healthcare, finance, and security. It also aids in compliance with data protection regulations like GDPR.
🏭 Use Cases Across Industries
🚘 Autonomous Vehicles
Self-driving cars generate terabytes of data daily. Edge AI allows them to interpret surroundings, predict behavior, and navigate safely — all in real time, without relying on cloud latency.
🏥 Healthcare and Remote Monitoring
Wearables and diagnostic devices powered by Edge AI can detect anomalies like arrhythmias or blood sugar spikes instantly and alert healthcare providers, improving response time and patient outcomes.
🏗️ Smart Manufacturing
Factories leverage Edge AI for predictive maintenance, visual inspection, robotics control, and environmental monitoring. This local intelligence helps maintain uptime and reduce costly disruptions.
🎥 Security and Surveillance
Edge-enabled cameras can detect suspicious activity, identify individuals, and trigger alerts or actions in real time, even without cloud access.
🌾 Agriculture and Environmental Monitoring
Drones and IoT sensors with Edge AI support precision farming by identifying crop diseases, monitoring soil conditions, or tracking wildlife behavior — all on-site and in real time.
🔧 Enablers of Edge AI in Decentralized Systems
Several technological advances are making Edge AI more viable and powerful:
💻 Lightweight AI Models
AI frameworks like TensorFlow Lite, ONNX Runtime, and PyTorch Mobile allow for efficient, on-device inference without heavy computational loads.
⚙️ Specialized Hardware
Edge devices are increasingly equipped with AI-specific hardware accelerators — like Google Coral, NVIDIA Jetson, and Intel Movidius — to deliver high-speed performance with low power consumption.
📶 5G and Next-Gen Networks
High-bandwidth, low-latency communication enables better synchronization between distributed nodes, though Edge AI can operate autonomously when needed.
🤖 Federated Learning
Federated learning enables decentralized training of models across multiple edge devices without sharing raw data — enhancing both privacy and learning efficiency.
🔐 Security and Ethical Considerations
With great power comes great responsibility. As Edge AI systems grow, so do concerns around security, bias, and transparency.
- Security: Edge devices are often physically accessible and can be targeted for tampering. Securing firmware, encrypting data, and enabling secure boot mechanisms are essential.
- Bias and Fairness: On-device models must be trained with diverse data to ensure fair, unbiased decision-making across use cases.
- Explainability: Interpreting AI behavior on low-power devices is challenging but necessary, especially for regulated industries.
🚀 Future Outlook: What’s Next for Edge AI?
The convergence of Edge AI and decentralized computing is still evolving, but the trajectory is clear. In the near future, expect to see:
- AI-powered microgrids, enabling smarter energy management at the edge.
- Collaborative AI agents across drones, robots, and IoT nodes working together to solve complex problems.
- Distributed AI marketplaces, where edge devices participate in shared intelligence networks.
- Self-organizing systems in smart cities and disaster response.
Ultimately, Edge AI will not just enhance existing systems — it will redefine them.
🧠 Final Thoughts
The shift toward decentralized, real-time computing is inevitable — and Edge AI is leading the way. By processing data where it’s created, enabling autonomous decisions, and reducing reliance on centralized infrastructures, Edge AI is unlocking new possibilities across every industry.
Whether you’re building autonomous vehicles, monitoring remote pipelines, or designing intelligent retail environments, Edge AI will be at the heart of your real-time, decentralized solution.
Now is the time to rethink the edge — not as a peripheral add-on, but as the center of intelligent action.
Follow us for more Updates